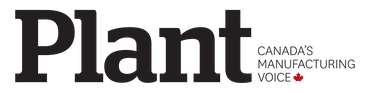
The future of plant asset management: How AI is leading the way
By Treena Hein
Operations ManufacturingIndustry experts agree that companies that do not implement AI to harness their asset-generated data will eventually either be put out of business by competitors or acquired.
The goals of plant asset management — among them improved efficiency, resource allocation and strategic planning—have not changed over time, but with the application of artificial intelligence (AI), these goals are much easier to reach.
And with this technology, unprecedented new goals are possible. It’s no exaggeration to say that AI is currently transforming how companies manage their assets (and entire plants), providing unsurpassed efficiency improvements in predictive maintenance, asset lifespan decision-making, automated optimization of asset operation and more.
Indeed, industry experts agree that companies that do not implement AI to harness their asset-generated data will eventually either be put out of business by competitors or acquired. Some companies have taken the plunge already, the so-called early adopters.
While Ray Morcos, CEO at Australia-based enterprise asset management firm Mainpac, describes the use of AI-level plant asset management (AM) as “still emerging” globally and “in the early stages,” he characterizes it has having “a growth rate that’s significant.” Morcos puts the estimated adoption rate among large industrial plants at 10-20 per cent globally at this point, but adds, “in North America and Europe, the penetration might be slightly higher.”
For her part, Stacey Jones, ABB’s global asset performance management portfolio lead, puts the percentage of AI-assisted AM globally at less than 10. Looking back, Jones observes that it was only a decade ago that in asset management, all plant operators and heads of maintenance could do was track data from asset sensors in a spreadsheet.
“They couldn’t scale that,” she explains, “and a team could only look at a limited number of critical assets to try and detect issues. Maintenance was mainly done according to manufacturer recommendations. With AI, we can scale asset management across a plant, and investigate mechanical or process issues much more efficiently.” She considers the release of user-friendly platforms such as ChatGTP and Microsoft Copilot to be significant drivers in AI adoption in manufacturing.
Managing asset lifespans, she adds, is another area where plant managers in the past have been only able to use their experience and simple calculations, but now is an area where AI can take analysis to new depths. The use of AI in asset management, Jones says, is all about AI lending its powerful and rapid mathematical strengths to support the human ability to make decisions.
Moving to the next level
Due to those strengths, Morcos characterizes AI-level AM as “a substantial leap” in comparison to the standard AM monitoring/reporting/recommendation software that’s been in place for years. He says the key difference between the two lies in AI’s ability to analyze vast amounts of both historical and real-time data, which significantly enhances predictive maintenance and decision-making. In other words, “traditional software is often rule-based, reactive and dependent on static reports,” Morcos explains, “while AI can dynamically interpret patterns, make proactive recommendations and adapt to new information as it becomes available.”
Morcos further notes that the pattern recognition capabilities of AI-level AM systems are superior to traditional AM software, offering an entirely new level of insight. Both the accuracy of the analysis and its depth are more advanced, with AI capable of identifying subtle trends that may be missed by traditional AM software systems (although some of these systems can highlight trends based on historical data).
Overall, Morcos asserts that these deeper insights that AI’s real-time analysis and predictive capabilities “can save plants millions of dollars annually,” through things like “preventing costly failures and optimizing resource allocation.”
In an AM context, AI can also be used to provide more accurate and reliable asset parts inventories, ensuring the need for urgent parts is better met, identifying obsolete inventory and so on. AI can also design automation systems for a facility, says Clinton Schneider, VP, innovation and technology for Emerson’s final control business, producing various asset configuration layouts and interconnections. Plant-wide, AI can also detect ambient temperature changes and as appropriate, adjust asset operation levels accordingly.

Five years ago, Emerson’s AMS Optics and augmented/virtual reality guided users to locations and provided manuals for plant asset maintenance. Now, with generative AI, the copilot model offers the same information, but users can interact in natural language, asking questions and receiving responses.
Integrating AI in AM
ABB, a global technology company that specializes in electrification and automation solutions, has had projects exploring AI use in AM and plant management since about 2014. Benedikt Schmidt, leader of ABB’s digital applications process control platform, is currently working with colleagues in Germany on what they call Augmented Operator plant-assistance systems.
Schmidt explains that among the many benefits of these systems in asset and overall plant management is the significant benefit of continuity. “When plant managers retire or leave—and these people are really experts on their plants, they have been completely in tune with their plants for many years—you have a gap,” he notes. “There are a number of areas that AI can be useful to bridge that gap, given the complexity AM and plant management.”
He and his colleagues are currently piloting an Augmented Operator-related ABB AI system called Knowledge Extractor. “It is capable of analysing hundreds of millions of data points related to the production process in terms of all relevant elements,” says Schmidt. “Analysis of data from the production process and the assets, the history of the plant and many other sources of data give an understanding of abnormal or unforeseen operations and countermeasures.”
That is, the system also enables comparison of the current plant state to the past, identifying, for example, that an exceptional situation currently occurring also happened 30 times in the last year, five times in the previous year and never before that. “Then you can narrow in on the operation and the equipment that’s causing the issue,” says Schmidt. “This helps the plant staff in making good decisions. It’s often hard to understand how something is building up, where in the process something is going wrong, so we work on sensing that early. And we can connect that to the knowledge bases of the plant to see how people in the past dealt with a similar situation, to better support current staff.”
To help deal with asset issues, Augmented Operator also offers a ‘What if’ function where you can ask, for example, what will happen if a certain valve is closed, and the AI will very quickly list the consequences.
Schneider also notes the capability of AI ‘copilots’ to allow all plant personnel to access even the most complex value-add capabilities and operate as subject matter experts, with “the ability to interact using natural language.”
He explains that five years ago, Emerson (using augmented reality/virtual reality) enabled users of its AMS Optics system to be guided to a location and assisted them in pulling up manuals and instructions to maintain plant assets. “Now with generative AI,” he says, “you have the copilot model that you can use to access those same manuals or instructions, but you can do it in natural language, asking questions and getting responses versus searching or reading manuals.”
Schneider believes that these new abilities of AI to work in natural language will be “transformative” in driving AM productivity.
“AI can ingest all the documentation, support interactions, logbooks and application notes to create a product chatbot [with] vast knowledge to answer questions about the product,” he says. He adds that “using AI to analyze engineering specifications including product guides and user manuals, Emerson has developed automated responses for customer inquiries that historically would have required engaging with an engineer.”
According to Morcos, the rollout of AI-driven chatbots and virtual assistants is already happening in many industries to assist maintenance teams to quickly access information, troubleshoot issues and make decisions on the fly. He says this trend is likely to accelerate in the next few years as AI assistants become more sophisticated and integrated into existing AM platforms.
Assets managing assets
Are we headed into a future where AI systems make autonomous decisions regarding asset and plant management instead of supporting human decision making? Or for that matter, a future where there are AI-powered robot assets that can do their own asset inspections and diagnostics (and even repairs) without human intervention?
Schmidt and Jones note that some of these systems are already in development. For example, there are mobile data-gathering units that can take video of the level of corrosion on assets, with software that will be able to carry out reliable analysis of the images and determine the best course of action.
There is already a robot asset that has successfully detected gas leaks. In 2023, Boston Dynamics’ robot dog Spot conducted an autonomous inspection of a 40-acre midstream gas plant. Equipped with visual and thermal cameras, a MUVE C360 gas-detection sensor and more, Spot was able to “sniff out” a potentially hazardous gas leak at a midstream gas plant during an autonomous inspection.
However, everyone in the industry agrees that if autonomous asset management—and autonomous assets such as Spot—are to become widespread, they not only have to be safe and as efficient (or more efficient) than human workers, but also cost-effective.
In addition, Schneider notes that future extension of AI in asset management poses challenges “including the right format and context for data quality, storage, usage, privacy, interactivity and access.” AI-level asset management systems must also be carefully assessed for potentially poor, incorrect, biased or harmful outputs, he says, and must be securely and effectively integrated with existing processes and systems.
Like any technology in development, AI has its share of potential challenges on the road ahead. But with the significant benefits that AI provides in managing assets and given that early adopters are seemingly enjoying solid success (not to mention AI is now engaged in improving its own capabilities through writing code itself), the industry can expect that these hurdles will be overcome, especially as AI continues to grow in power.
Print this page
Advertisement
<